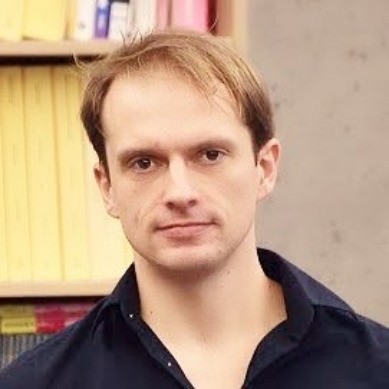
Ben Cardoen
Computational scientist designing novel weakly supervised reconstruction and detection algorithms in superresolution microscopy.
I design novel weakly supervised interaction analysis algorithms on volumetric and point cloud data from superresolution microscopy.
I leverage my background in parallel and distributed discrete event simulators PDEVS
(B.Sc.), meta- and hyperheuristics, and symbolic regression (M. Sc.) in my current research.
My current focus is extending probabilistic learning algorithms to reconstruct the interaction
between proteins
and organelles
, often below
the limit of the acquisition, in multichannel superresolution microscopy
on scales from 100 to 10nm.
The size of the data necessitates that my algorithms are scalable
, leveraging both shared memory parallelism and distributed computing, often on SLURM
based supercomputers.
I extend weakly supervised learning
using belief theory
to support nested labels, recover equilibria transitions, and leverage closed form upper and lower boundaries to the probability of each object supporting a subset of the labels.
Labels can in turn be genomic
, relate to viral
infection, drug treatment
, or environmental stressors to the cell
, or a combination of both.
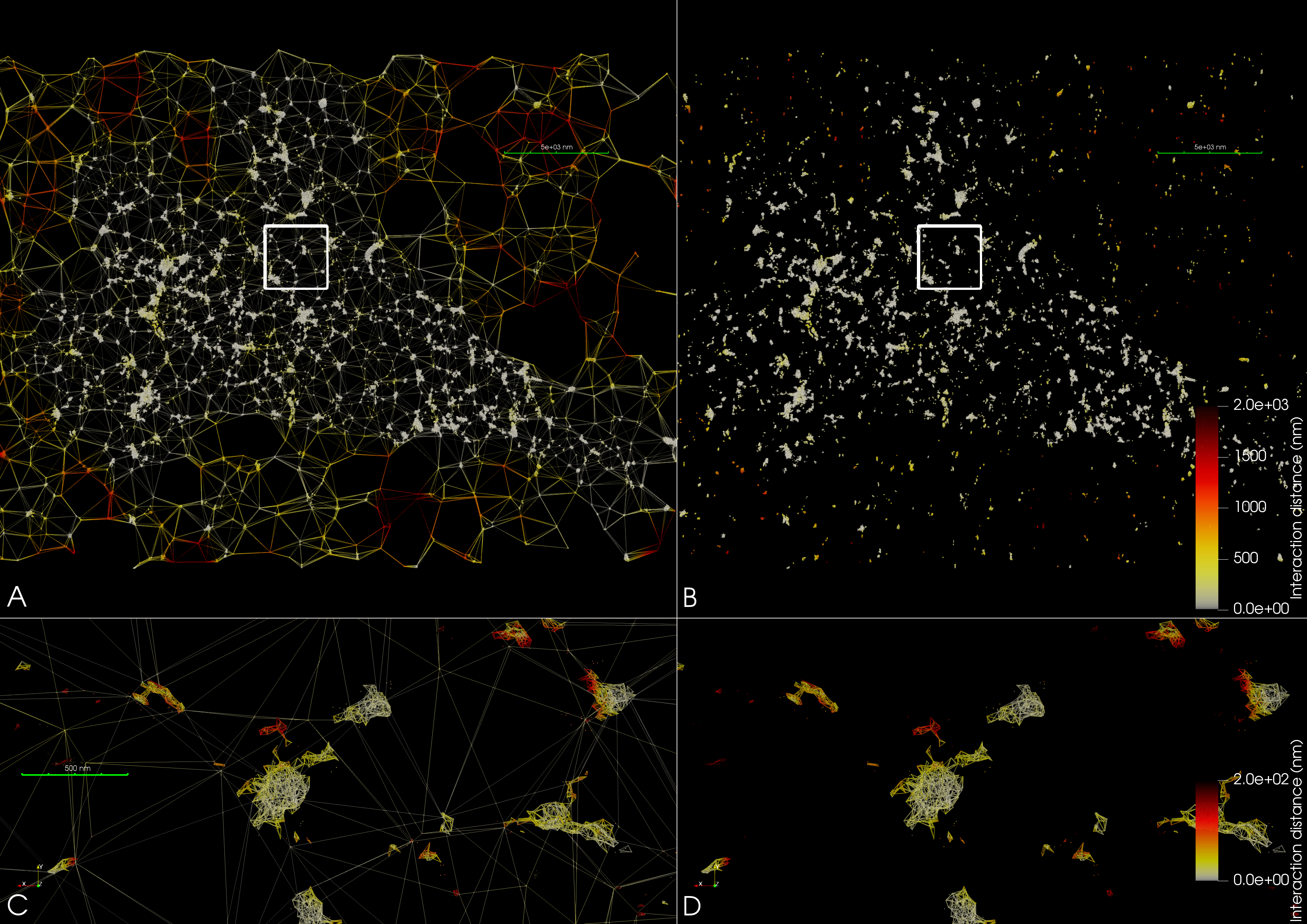
The 3D rendering shows a discrete vectorfield
interaction graph between two SMLM
point cloud datasets, see publications for the relevant papers.
PDFs of my publications are publicly available , while a more in depth description of the projects is listed here.
I post on both research, philosophy, and technical problems in blog posts.